SOS-PVCase: A machine learning optimized lignin peroxidase with polyvinyl chloride (PVC) degrading properties
(1) Millburn High School, (2) Harvard University
https://doi.org/10.59720/23-167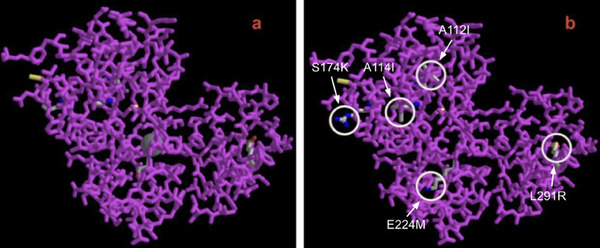
Plastic accumulating in landfills poses a threat to wildlife ecosystems and contributes to the production of harmful greenhouse gases. Polyvinyl chloride (PVC) accounts for 12% of plastic manufactured worldwide, and currently, 79% of post-consumer PVC ends up in landfills. Enzymatic degradation of plastic polymers into reusable monomers provides a green and scalable solution to this expanding problem. However, the application of PVC degrading peroxidases in real-world environments is impaired by their lack of stability and solubility. Fungal lignin peroxidase (E.C 1.11.1.14), an enzyme expressed by Phanerochaete chrysosporium, has previously been identified to have PVC degradation properties but, nevertheless, is hindered by the same constraints. In our study, we hypothesized that mutations in the primary structure of lignin peroxidase can improve the stability and solubility of the enzyme. To test our hypothesis, we utilized publicly available machine learning models to pinpoint stabilizing and solubilizing mutations of lignin peroxidase. The optimized mutant protein (SOS-PVCase: stable, optimized, soluble) contains five amino acid substitutions (A112I, A114I, S174K, E224M, L291R) which collectively improve stability by 18.6% and solubility by 10.2% compared to the wild-type enzyme, supporting our hypothesis that some mutations can enhance the protein’s stability and solubility. This is important, as it allows PVC recycling pathways to be accelerated and made more economical. Furthermore, this suggests the potential of utilizing machine learning for the purpose of protein optimization.
This article has been tagged with: