Evaluating TensorFlow image classification in classifying proton collision images for particle colliders
(1) Huntington Beach High School, Huntington Beach, California
* These authors made equal contributions
https://doi.org/10.59720/23-013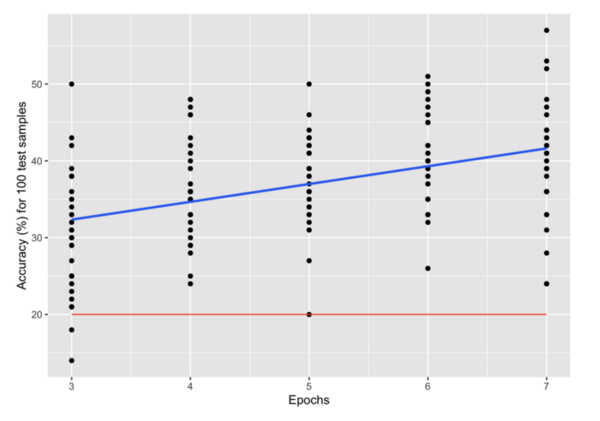
The Large Hadron Collider at the European Organization for Nuclear Research (CERN) is planned to increase the number of proton collisions 10-fold by 2025. To keep up with this rapid pace, novel and more efficient particle collision classification methods must be developed, which many physicists believe can be achieved via machine learning. Since particle collisions and trajectories are mapped onto images, we chose TensorFlow’s image classification model to analyze and predict particle classes from these collision images. We aimed to evaluate the accuracy at which image classification could correctly identify a particle’s category from its trajectory image. We hypothesized that image classification would improve its accuracy with larger training datasets and more training epochs. To test our hypotheses, we randomly partitioned 100 images for testing and reserved 3,400 for training. We ran numerous trials using different training set sizes, different training epoch values, and binary classification. Our results show that the model’s accuracy increases with more training samples, and it increases its accuracy and consistency with more training epochs. In binary classification, the model distinguishes the electron class the best, with a 95.2% mean accuracy. We conclude that image classification has remarkable implementation potential in classifying particle collision outcomes because of its ability to improve with training sample size and in binary classification. Our work highlights one method collision classification algorithms should utilize, which can work effectively with the increased data output by particle colliders and give physicists a vital tool in accelerating their field.
This article has been tagged with: