Using data science along with machine learning to determine the ARIMA model’s ability to adjust to irregularities in the dataset
(1) BASIS Independent Silicon Valley, (2) Department of Computer Science, Aspiring Scholars Directed Research Program
https://doi.org/10.59720/21-048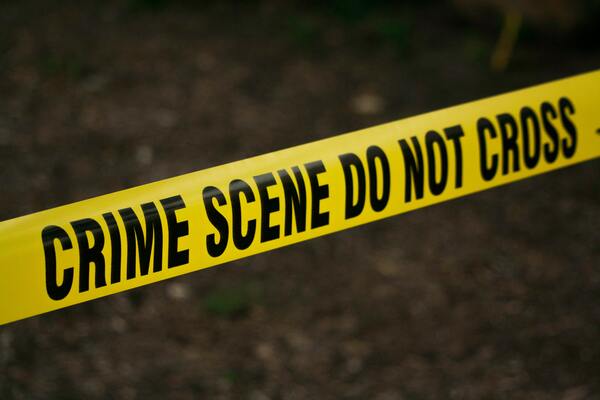
Auto-Regressive Integrated Moving Average (ARIMA) models are known for their influence and application on time series data. This statistical analysis model uses time series data to depict future trends or values: a key contributor to crime mapping algorithms. With crime being a concerning topic in many urban areas like Chicago and Oakland, crime mapping algorithms have become a topic of discussion. However, the models may not function to their true potential when analyzing data with many different patterns. In order to determine the potential of ARIMA models, our research will test the model on irregularities in the data. Our team hypothesizes that the ARIMA model will be able to adapt to the different irregularities in the data that do not correspond to a certain trend or pattern. Using crime theft data and an ARIMA model, we determined the results of the ARIMA model’s forecast and how the accuracy differed on different days with irregularities in crime. For comparison purposes, we compared the model’s error when implemented on stationary data. Our results show that the model is accurate with a margin of error at least 25 cases per day when there were 250 cases per day on average. These findings will help law enforcement systems who are focused on crime suppression and help future researchers that are interested in utilizing ARIMA models to reach its true potential.
This article has been tagged with: