Hybrid Quantum-Classical Generative Adversarial Network for synthesizing chemically feasible molecules
(1) BASIS Independent Silicon Valley, San Jose, CA, (2) Sir Winston Churchill Secondary School, Vancouver, British Columbia, (3) Archbishop Mitty High School, San Jose, CA, (4) The Episcopal Academy, Newton Square, PA, (5) Monta Vista High School, Cupertino, CA, (6) Department of Computer Science and Engineering, Aspiring Scholars Directed Research Program, Fremont, CA
https://doi.org/10.59720/22-143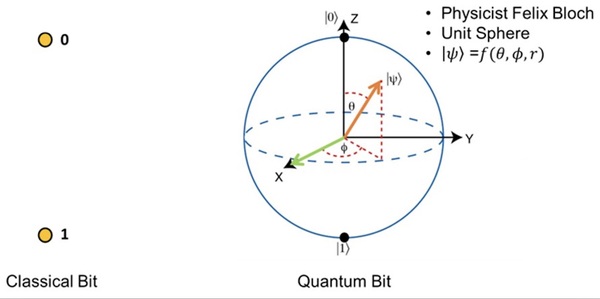
Current drug discovery processes can cost billions of dollars and usually take five to ten years. People have been researching and implementing various computational approaches to search for molecules and compounds from the chemical space, which can be on the order of 1060 molecules. One solution involves deep generative models, which are artificial intelligence models that learn from nonlinear data by modeling the probability distribution of chemical structures and creating similar data points from the trends it identifies. These generative models can extract salient features that characterize the molecules. However, they often suffer from increased time complexity. Aiming for faster runtime and greater robustness when analyzing high-dimensional data, we designed and implemented a Hybrid Quantum-Classical Generative Adversarial Network (QGAN) to synthesize molecules. There are two parts in the QGAN: a quantum generator that creates molecules based on the probability distributions of likely combinations and a classical discriminator that differentiates real molecules from the generated ones. We hypothesized that a quantum generator would be more impactful, offering a runtime speedup and increasing generated molecule possibilities, because we could use quantum mechanical phenomena superposition, entanglement, and interference to analyze more atom-bond combinations than a classical generator. The PyTorch-PennyLane implementation of the QGAN generated seven chemically stable molecules out of 300, a 2.3% success rate. Although the QGAN is a work in progress, it has demonstrated a path towards more efficient drug development, which would accelerate the development of medicines and reduce costs for the whole R&D process.
This article has been tagged with: