Using two-stage deep learning to assist the visually impaired with currency differentiation
(1) The Harker School, (2) AIClub Research Institute
https://doi.org/10.59720/23-045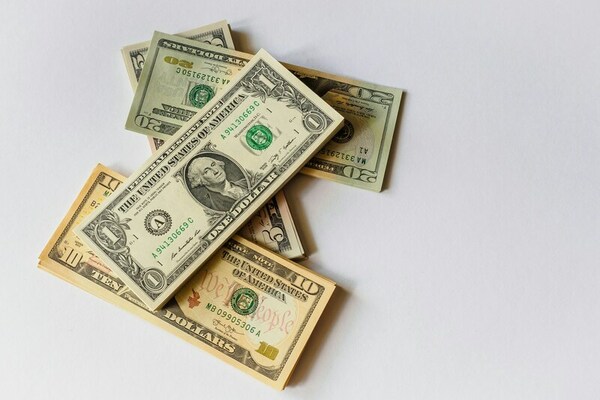
Approximately 2.2 billion individuals across the world possess varying degrees of visual impairments, and they are challenged with a plethora of difficulties in their day-to-day experiences. In this study, we explored the application of deep learning algorithms to aid individuals with visual impairments in recognizing United States (US) currency. To address real-world issues such as damaged bills and background variations, we created a custom dataset specifically tailored for this task. We hypothesized that the utilization of a two-stage deep learning model would be more efficient and accurate than a single-stage deep learning model to address this problem. To test this hypothesis, we used the aforementioned artificial intelligence (AI) models trained on a custom dataset specifically designed to address real-world challenges to solve this problem. We chose the MobileNetV2 architecture for our experiments owing to its small size and potential for deployment on a mobile device. We also analyzed the importance of choosing optimal model hyperparameters in the context of this dataset. Through our experiments and choosing the models with the best validation accuracies, we achieved a test accuracy of 89% with a single-stage AI and a test accuracy of 83% with a two-stage AI. Our results, however, showed that a multi-level deep learning model did not provide any significant advantage over a single-level AI.
This article has been tagged with: