Identifying factors, such as low sleep quality, that predict suicidal thoughts using machine learning
(1) Branham High School, (2) Department of Psychology, Princeton University
https://doi.org/10.59720/23-999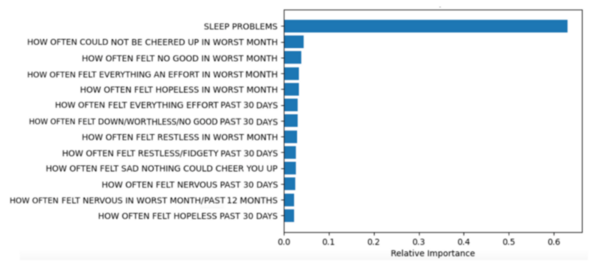
Every year, around 800,000 people die by suicide worldwide, and it is the second leading cause of death for individuals aged 15-24 years. The National Survey on Drug Use and Health (NSDUH) is conducted annually to ask the general United States population aged 12 years and older questions about mental health, substance use, and suicidal thoughts. Some of the factors the survey considers are substance use, including need for treatment and disorders, and mental health topics, including major depressive episodes, suicidal ideation and attempts, and mental illness. In our research, we sought to identify associations between suicidal ideation and relevant variables, such as sleep quality, hopelessness, and anxious behavior, by analyzing survey responses from the 2020 NSDUH. Due to the density of the survey and no obvious direct relationship between any variables with suicidal ideation, through our research we aimed to clearly find and display any association. We hypothesized that frequent anxious thoughts and behavior (such as fidgeting and restlessness), feelings of sadness, and/or low sleep quality would be the factors most predictive of having suicidal thoughts. Using a random forest classifier, we found that sleep problems were highly predictive of suicidal ideation. Professionals and clinicians should keep these findings in mind when developing suicide prevention efforts, such as identifying and supporting people potentially at risk, intervening to improve sleep quality, and teaching coping skills.
This article has been tagged with: