Machine learning for the diagnosis of malaria: a pilot study of transfer learning techniques
(1) Horizon Academic Research Program, Jersey City, NJ, USA; Computer Science, Grainger College of Engineering, University of Illinois, Urbana-Champaign, IL, USA, (2) Horizon Academic Research Program, Jersey City, NJ, USA; Department of Computer Science, University of Chicago, Chicago, IL, USA
https://doi.org/10.59720/21-015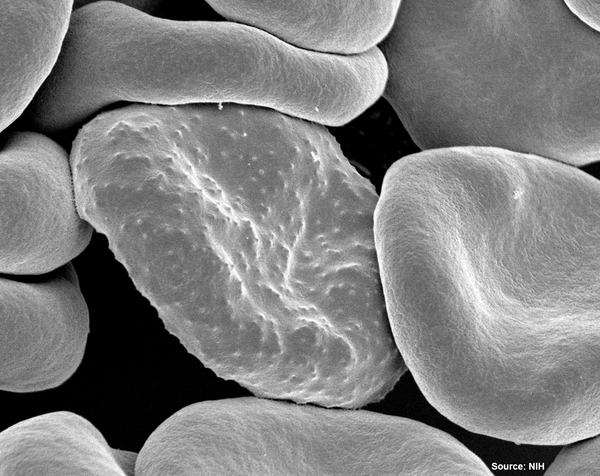
Despite being treatable and preventable, malaria continues to affect millions of people worldwide. A major hindrance to the disease’s eradication is difficulty with testing, and attempts have already been made to shift towards more automated testing methods rather than older testing technologies such as light microscopy and rapid diagnostic tests. Machine learning models have shown promise in diagnosing malaria, but prior machine learning algorithms have been contingent upon the availability of clean and relevant data. Furthermore, there are multiple species of malaria that may require different treatments, often resulting in fewer comparative images of individual malarial subtypes on blood slides of a given patient population. In this pilot study, we tested a machine learning technique called transfer learning to reduce the error caused by lesser amounts of available data. By training the algorithm on a known (but not necessarily related) dataset and then re-training on malaria datasets, we were able to significantly increase diagnostic accuracy. Specifically, using transfer learning to prepare the algorithm on a completely unrelated database before applying to malaria slide interpretation, our diagnostic accuracy improved from 65.5% to 80.7% in a small dataset of malaria blood slides, and from 95.5% to 98.0% in a much larger malarial dataset. Moving forward, these types of experiments will help develop a smartphone application to increase the opportunity to diagnose malaria in places where resources are too limited to permit standard diagnostic techniques.
This article has been tagged with: